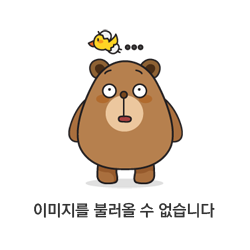
CART(Classification and Regression Tree)
가장 많이 쓰는 기법
C4.5 & C5.0
CART와 다르게 node에서 다지분리(Multiple Split)이 가능
CHADID(Chi-squared Automatic Interaction Detection)
범주형 변수에 적용 가능
1. 4.1 의사결정 트리(Decision Tree)
의사결정트리 방식은 나무(Tree)구조 형태로 분류 결과를 도출
1.0.1. (1) party 패키지 이용 분류분석
조건부 추론 나무
CART기법으로 구현한 의사결정나무의 문제점
1) 통계적 유의성에 대한 판단없이 노드를 분할하는데 대한 과적합(Overfitting) 발생 문제.
2) 다양한 값으로 분할 가능한 변수가 다른 변수에 비해 선호되는 현상
이 문제점을 해결하는 조건부 추론 나무(Conditional Inference Tree).
party패키지의 ctree()함수 이용
실습 (의사결정 트리 생성: ctree()함수 이용)
<r />
1단계: party패키지 설치
install.packages("party")
library(party)
2단계: airquality 데이터셋 로딩
library(datasets)
str(airquality)
datasets 패키지
3단계: formula생성
formula <- Temp ~ Solar.R + Wind + Ozone
4단계: 분류모델 생성 – formula를 이용하여 분류모델 생성
air_ctree <- ctree(formula, data = airquality)
air_ctree
7) Ozone <= 65, criterion=0.971, statistic = 6.691
(1) (2) (3) (4)
첫번째: 반응변수(종속변수)에 대해서 설명변수(독립변수)가 영향을 미치는 중요 변수의 척도.
수치가 작을수록 영향을 미치는 정도가 높고, 순서는 분기되는 순서를 의미
두번째: 의사결정 트리의 노드명
세번째: 노드이 분기기준(criterion)이 되는 수치.
네번째: 반응변수(종속변수)의 통계량(statistic).
*마지막 노드이거나 또 다른 분기 기준이 있는 경우에는 세번째와 네 번째 수치는 표시되지 않는다.
5단계: 분류분석 결과
plot(air_ctree)
실습 (학습데이터와 검정데이터 샘플링으로 분류분석 수행)
<r />
1단계: 학습데이터와 검정데이터 샘플링
#set.seed(1234)
idx <- sample(1:nrow(iris), nrow(iris) * 0.7)
train <- iris[idx, ]
test <- iris[-idx, ]
2단계: formula생성
formula <- Species ~ Sepal.Length + Sepal.Width + Petal.Length + Petal.Width
3단계: 학습데이터 이용 분류모델 생성
iris_ctree <- ctree(formula, data = train)
iris_ctree
ctree 함수
https://www.rdocumentation.org/packages/partykit/versions/1.2-13/topics/ctree
4단계: 분류모델 플로팅
4-1단계: 간단한 형식으로 시각화
plot(iris_ctree, type = "simple")
4-2단계: 의사결정 트리로 결과 플로팅
plot(iris_ctree)
5단계: 분류모델 평가
5-1단계: 모델의 예측치 생성과 혼돈 매트릭스 생성
pred <- predict(iris_ctree, test)
table(pred, test$Species)
5-2단계: 분류 정확도
(14 + 16 + 13) / nrow(test)
실습 (K겹 교차 검정 샘플링으로 분류 분석하기)
<r />
1단계: k겹 교차 검정을 위한 샘플링
library(cvTools)
cross <- cvFolds(nrow(iris), K = 3, R = 2)
2단계: K겹 교차 검정 데이터 보기
str(cross)
cross
length(cross$which)
dim(cross$subsets)
table(cross$which)
3단계: K겹 교차 검정 수행
R = 1:2
K = 1:3
CNT = 0
ACC <- numeric()
for(r in R) {
cat('\n R = ', r, '\n')
for(k in K) {
datas_ids <- cross$subsets[cross$which == k, r]
test <- iris[datas_ids, ]
cat('test : ', nrow(test), '\n')
formual <- Species ~ .
train <- iris[-datas_ids, ]
cat('train : ', nrow(train), '\n')
model <- ctree(Species ~ ., data = train)
pred <- predict(model, test)
t <- table(pred, test$Species)
print(t)
CNT <- CNT + 1
ACC[CNT] <- (t[1, 1] + t[2, 2] + t[3, 3]) / sum(t)
}
}
CNT
4단계: 교차 검정 모델 평가
ACC
length(ACC)
result_acc <- mean(ACC, na.rm = T)
result_acc
실습 (고속도로 주행거리에 미치는 영향변수 보기)
<r />
1단계: 패키지 설치 및 로딩
library(ggplot2)
data(mpg)
ggplot2패키지
2단계: 학습데이터와 검정데이터 생성
t <- sample(1:nrow(mpg), 120)
train <- mpg[-t, ]
test <- mpg[t, ]
dim(train)
dim(test)
3단계: formula작성과 분류모델 생성
test$drv <- factor(test$drv)
formula <- hwy ~ displ + cyl + drv
tree_model <- ctree(formula, data = test)
plot(tree_model)
실습 (Adultuci 데이터 셋을 이용한 분류분석)
<r />
1단계: 패키지 설치 및 데이터 셋 구조 보기
library(arules)
data(AdultUCI)
str(AdultUCI)
names(AdultUCI)
arules패키지
데이터셋 AdultUCI데이터 셋
https://www.rdocumentation.org/packages/arules/versions/1.6-8/topics/Adult
2단계: 데이터 샘플링
set.seed(1234)
choice <- sample(1:nrow(AdultUCI), 10000)
choice
adult.df <- AdultUCI[choice, ]
str(adult.df)
3단계: 변수 추출 및 데이터프레임 생성
3-1단계: 변수 추출
capital <- adult.df$`capital-gain`
hours <- adult.df$`hours-per-week`
education <- adult.df$`education-num`
race <- adult.df$race
age <- adult.df$age
income <- adult.df$income
3-2단계: 데이터프레임 생성
adult_df <- data.frame(capital = capital, age = age, race = race,
hours = hours, education = education, income = income)
str(adult_df)
4단계: formula생성 – 자본이득(capita)에 영향을 미치는 변수
formula <- capital ~ income + education + hours + race + age
5단계: 분류모델 생성 및 예측
adult_ctree <- ctree(formula, data = adult_df)
adult_ctree
6단계: 분류모델 플로팅
plot(adult_ctree)
7단계: 자본이득(capital) 요약 통계량 보기
adultResult <- subset(adult_df,adult_df$income == 'large' & adult_df$education > 14)
length(adultResult$education)
summary(adultResult$capital)
boxplot(adultResult$capital)
실습2
<r />
# 조건부추론나무
install.packages("party")
library(party)
# sampling
str(iris)
set.seed(1000)
sampnum <- sample(2, nrow(iris), replace=TRUE, prob=c(0.7,0.3))
sampnum
# training & testing data 구분
trData <- iris[sampnum==1,]
head(trData)
teData <- iris[sampnum == 2, ]
head(teData)
shortvar <- Species~Sepal.Length+Sepal.Width+Petal.Length+Petal.Width
# 학습
citreeResult <- ctree(shortvar, data=trData)
# 에측값과 실제값 비교
table(predict(citreeResult), trData$Species)
citreeResult2 <- ctree(shortvar, data=teData)
# 테스트 데이터를 이용하여 분류
forcasted2 <- predict(citreeResult2, data=teData)
# forcasted
# teData$Species
# 예측결과와 실제값 비교
table(forcasted2, teData$Species)
#시각화
plot(citreeResult2)
결과 해석
종(Species) 판단
Petal.Length <= 1.9 : setosa로 판단
Petal.Length > 1.9 & Petal.Width <= 1.6 : versicolor로 판단
나머지: virginica 로 판단
(2) rpart패키지 이용 분류분석
CART(Classification and Regression Tree)
rpart 패키지
실습 (rpart()함수를 이용한 의사결정 트리 생성)
rpart()함수
형식: rpart(반응변수 ~ 설명변수, data)
https://www.rdocumentation.org/packages/rpart/versions/4.1-15/topics/rpart
<r />
1단계: 패키지 설치 및 로딩
install.packages("rpart")
library(rpart)
install.packages("rpart.plot")
library(rpart.plot)
rpart패키지
2단계: 데이터 로딩
data(iris)
3단계: rpart()함수를 이용한 분류분석
rpart_model <- rpart(Species ~ ., data = iris)
rpart_model
4단계: 분류분석 시각화
rpart.plot(rpart_model)
rpart.plot()함수
https://www.rdocumentation.org/packages/rpart.plot/versions/3.0.9/topics/rpart.plot
실습 (날씨 데이터를 이용하여 비(rain)유무 예측
<r />
1단계: 데이터 가져오기
weather = read.csv("C:/Rwork/weather.csv", header = TRUE)
2단계: 데이터 특성 보기
str(weather)
head(weather)
데이터셋 (weather 데이터 셋)
366개 관측치, 15개의 변수
3단계: 분류분석 데이터 가져오기
weather.df <- rpart(RainTomorrow ~ ., data = weather[ , c(-1, -14)], cp = 0.01)
4단계: 분류분석 시각화
rpart.plot(weather.df)
5단계: 예측치 생성과 코딩 변경
5-1단계: 예측치 생성
weather_pred <- predict(weather.df, weather)
weather_pred
5-2단계: y의 범주로 코딩 변환
weather_pred2 <- ifelse(weather_pred[ , 2] >= 0.5, 'Yes', 'No')
6단계: 모델 평가
table(weather_pred2, weather$RainTomorrow)
(278 + 53) / nrow(weather)
실습2.
iris 데이터 사용
<r />
# 의사결정나무
# CART
install.packages("rpart")
library(rpart)
# 의사결정나무 생성
CARTTree <- rpart(Species~., data=iris)
CARTTree
# 의사결정나무 시각화
plot(CARTTree, margin=0.2)
text(CARTTree, cex=1)
# CARTTree를 이용하여 iris데이터 종 전체를 대상으로 예측
predict(CARTTree, newdata=iris, type="class")
# 결과 저장
predicted <- predict(CARTTree, newdata=iris, type="class")
# 예측정확도
sum(predicted == iris$Species) / NROW(predicted)
# 실제값과 예측값의 비교
real <- iris$Species
table(real, predicted)
결과해석
전체데이터 = 150
y의 값을 setosa로 하면 100개를 설명할 수 없음
각 종마다 확률은 0.3333으로 동일
Petal.Length가 2.45보다 작은 것이 50개 있고 y의 값을 setosa로 했을 때, 설명되지 않는 부분은 없음
Petal.Length가 2.45보다 크거나 같은 것이 100개. y의 값을 versicolor로 했을 때, 50개가 설명되지 않음
의사결정나무를 보면 species(종)인 setosa, versicolor, virginica를 식별하기 위하여 네개의 항목 중 Petal.Length와 Petal.Width만을 기준으로 사용. --> 두개의 기준으로 충분히 분리가 가능
결과로 부터 해석:
Petal.Length < 2.45 : setosa로 분류
Petal.Length > 2.45 & Petal_Width < 1.75 : vericolor로 분류
나머지: verginica로 분류
예측정확도: 96%
'데이터분석 > 머신러닝기반' 카테고리의 다른 글
서포트벡터머신(Support Vector Machine) (1) | 2022.12.12 |
---|---|
앙상블(Ensemble) (0) | 2022.12.12 |
다차원 척도법(Multi-Dimensional Scaling) (0) | 2022.12.12 |
변수 제거 (0) | 2022.12.12 |
베이지안(Baysian) (1) | 2022.12.12 |