728x90
https://arxiv.org/pdf/2208.12242v1.pdf
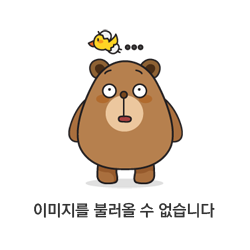
1. 초록
1.1. 몇개의 이미지로 주요 시각적 특징을 유지하면서 환경과 자연스러운 무수히 많은 이미지를 합성할 수 있다.
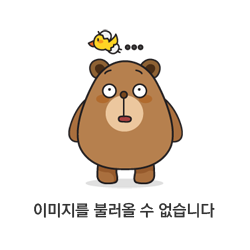
2. 방법
특정 개와 해당 클래스 이름의 이미지 3~5개가 입력이 되면 고유 식별자를 인코딩하는 개인화된 텍스트-이미지 모델을 생성한다. 그리고 추론시 고유 식별자를 다른문장에 삽입하여 다른 맥락에서 주제를 합성할 수 있다.
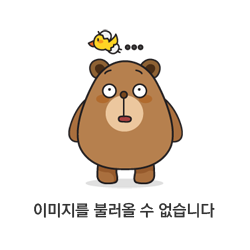
두 단계로 텍스트-이미지 확산을 미세조정 한다. 식별자와 주제가 속한 클래스의 이름과 병행하여 클래스별 사전 보존 손실(Prior Preservation Loss)을 적용한다. 텍스트 프롬프트에 클래스 이름을 삽입하여 대상 클래스에 속하는 다양한 인스턴스를 생성하도록 한다. 입력 이미지 세트에서 가져온 저해상도 및 고해상도 이미지 쌍으로 초고해상도 구성 요소를 미세 조정하여 피사체의 작은 세부 사항에 대해 높은 충실도를 유지한다.
3. 결과
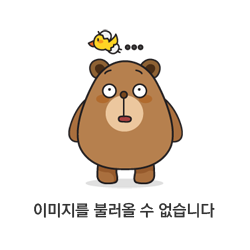
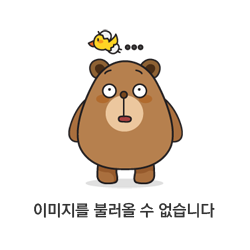
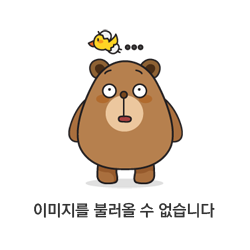
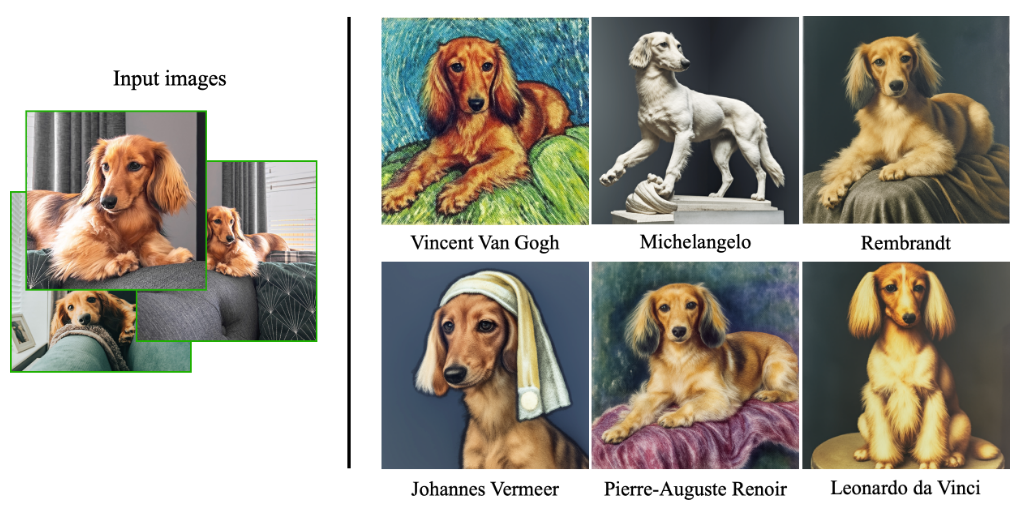
유명한 화과의 스타일로 개를 예술적으로 표현했다. 생성된 많은 포즈가 훈련세트에서는 볼 수 없었다. 화가의 스타일을 모방하는 것처럼 보인다.
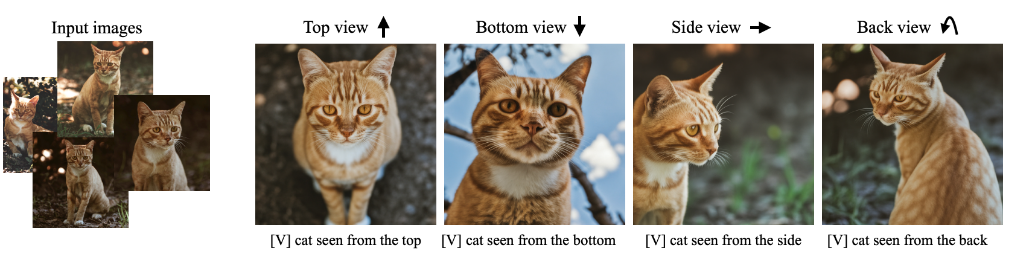
지정된 시점으로 이미지를 합성할수 있다. 생성된 포즈는 입력 포즈와 다르며, 포즈 변경에 따라 배경도 변경된다.
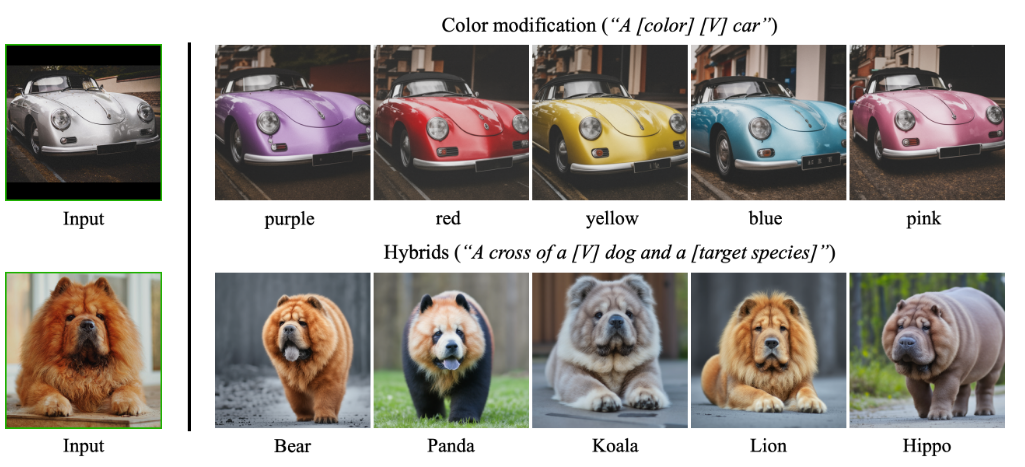
색상을 수정하고, 특정 개와 다른 동물간의 crosses된 이미지를 보여준다.
'영상처리 > 논문' 카테고리의 다른 글
Vision Transformer(ViT) : An Image Worth 16 x 16 Words (109) | 2023.05.15 |
---|---|
WeatherNet 다중 클래스 분류 논문 구현 아이디어 (192) | 2023.02.27 |
[ResNet]Deep Residual Learning for Image Recognition 리뷰 (0) | 2023.01.26 |
논문 읽는법 (10) | 2022.12.14 |
다중 클래스 아다부스트 알고리즘 (0) | 2022.12.13 |